Medical Image Segmentation With UNET
You can improve UNet training by using checkpoints, LR adjustments, label encoding, and seeing examples to make sure they work.
Save $20
Limited Time Offer
$30 USD
$10.00 USD
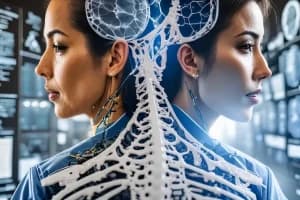
Project Outcomes
In this project, Medical Image Segmentation with UNET, our main outcomes are below,
- Implemented a new segmentation model for medical images, which increases diagnosis precision.
- Prove the efficiency of the proposed U-Net architecture with real medical images of MRI, CT, and X-ray images.
- Specifically facilitated the speed at which analysis and diagnosis were done by diminishing the amount of work done through the use of segmentation techniques in medical images.
- Improved diagnostic techniques due to highly sectioned anatomical and pathological accumulations.
- Assisted healthcare professionals in developing client/server-oriented prescriptions with exact and comprehensive segmentations.
- Data preshaping solutions for the consecutive processing and application of medical images as well as for the model training.
- Introduced Mean IoU and Accuracy as strategies for recognizing model progress and succeeded in its incorporation into the project.
- Helped in the visualization of the results of the segmentation to further clarify the model's predictions and their correlation with ground truth.
- Showed how AI can be utilized in enhancing medical imaging thus preparing the ground for further evolution in medicine diagnosis and management.
- Successfully implemented a U-Net model for medical image segmentation, improving diagnostic precision for conditions such as tumors and organ anomalies.
- Demonstrated the model's effectiveness in processing various types of medical images, including MRI, CT, and X-ray.
- Increased efficiency in the segmentation process, reducing the time taken for healthcare professionals to analyze medical images.
- Established a framework that can be adapted for other medical imaging applications, enhancing the flexibility of the model across various healthcare contexts.
- Contributed to the development of automated tools for faster and more accurate diagnosis, thereby potentially improving patient outcomes in clinical settings.