Graph-Enhanced Retrieval-Augmented Generation (GRAPH-RAG)
GraphRAG is a document retrieval system that combines vector search, knowledge graph traversal and LLMs for accurate, context-aware query responses.
$20 USD
$10.00 USD
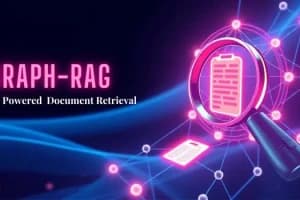
Project Outcomes
GraphRAG, an advanced retrieval-augmented generation system, offers several impactful outcomes across various industries:
-
Enhances document retrieval accuracy and relevance.
-
Improves question-answering systems by providing contextually relevant responses.
-
Enables hyper-personalized recommendation engines.
-
Strengthens fraud detection by uncovering hidden data patterns.
-
Accelerates biomedical research by mapping connections in medical studies.
-
Simplifies legal research by navigating complex legal data.
-
Optimizes supply chain management by analyzing and connecting data points.
-
Enhances customer support with more accurate, context-aware responses.
-
Facilitates scientific discovery by revealing hidden patterns in research.
-
Improves business intelligence for better strategic decision-making.