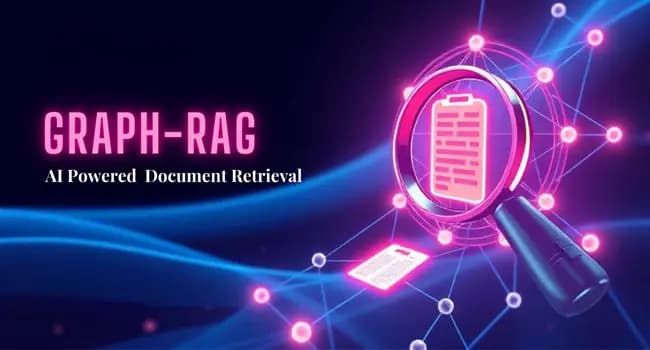
Graph-Enhanced Retrieval-Augmented Generation (GRAPH-RAG)
The GraphRAG project is designed to address the challenge of efficient document retrieval, processing and query answering by combining vector search, BM25 and knowledge graph traversal. The system allows for extracting contextually relevant information from large sets of documents and organizing it into a graph structure, enabling effective querying and response generation. By leveraging large language models (LLMs) and embedding models, GraphRAG offers a powerful solution for retrieving information, analyzing document content and generating accurate answers in real time.
Project Overview
The GraphRAG project represents a powerful system that uses vector search techniques and knowledge graph exploration to improve document searches and questioning processes. The system divides extensive documents into segments while creating embeddings, which get stored within a FAISS vector store for quick retrieval searches. A knowledge graph uses content similarity to establish document chunk relationships, which allows the chunks to connect into a comprehensive network. The system utilizes a QueryEngine to process queries by conducting graph traversals, which enable the retrieval of highly pertinent information. When the context falls short, the system uses a large language model (LLM) to create the missing answer. The Visualizer tool shows a graphical representation of graph traversal, allowing users to better understand the analysis process. Relevant context-based document understanding produces accurate results and supplies a strong method for textual information retrieval.
Prerequisites
- Python 3.6+: Required for running the project.
- Libraries: Install NetworkX, Matplotlib, FAISS, spaCy, OpenAIEmbeddings, NLTK, LangChain and PyPDFLoader.
- OpenAI API Key: Needed for embeddings and LLM interactions.
- Google Colab / Jupyter Notebook: Recommended for running the system.
- pip: For managing and installing dependencies.
- Basic Python Knowledge: Familiarity with Python libraries like pandas, numpy and scikit-learn.
Approach
GraphRAG retrieves documents and generates responses through processes of document processing, knowledge graph building and query expansion. It initially chunked the documents into small pieces through the use of a text splitter and then embedded them using OpenAIEmbeddings; these embeddings were later stored in a FAISS vector store for efficient similarity-based searching. A knowledge graph is then constructed where the node represents a document chunk and the edge follows the content similarity and shared concepts. The QueryEngine traverses this graph using modified Dijkstra's algorithm to expand the context around the query to ensure the visiting of the most relevant information, which gives the entire answer; otherwise, a final response would be generated by an LLM. The visualizer graphically represents the graph traversal to make the procedure transparent so that clicking any node can show how the system comes up with that answer. This contribution makes the process a comprehensive, rich contextual, yet efficient querying method; it is highly suitable for applications where deep document understanding is required.
Workflow and Methodology
Workflow of GraphRAG System
- Document Loading: Documents are loaded using PyPDFLoader.
- Document Processing: Documents are split into chunks embedded using OpenAIEmbeddings and stored in a FAISS vector store.
- Knowledge Graph Construction: A knowledge graph is built, connecting document chunks based on similarity and shared concepts.
- Query Handling: The QueryEngine retrieves relevant documents and traverses the knowledge graph to expand the context.
- Context Expansion: The engine explores the graph, updates the context and uses an LLM to generate an answer if needed.
- Visualization: The Visualizer shows the graph traversal, highlighting explored nodes and edges.
- Final Answer: The system provides the final answer to the query, generated either from the context or via the LLM.
Methodology
- Chunking and Embedding: The documents are chunked into smaller pieces and embedded into vectors, facilitating comparison and retrieval.
- Graph Construction: The initial document chunk embeddings and their cosine similarity are used to draw meaningful links in the knowledge graph.
- Query Expansion: The system expands the query context while traversing the graph and, thus, retrieves the most relevant information.
- LLM-based Answering: When the context is still insufficient, the system generates the answer with an LLM according to the expanded context.
- Graph Visualization: Matplotlib visualizing the traversal path ensures transparency for how the system arrived at the final answer.
Data Collection and Preparation
Data Collection
For this project, data is collected in the form of text documents, such as PDFs or other text-based formats. The documents can cover a variety of topics and in this case, a sample document named "Understanding_Climate_Change.pdf" is used. The goal is to extract meaningful information from these documents by splitting them into smaller, manageable chunks for further analysis. These documents are processed by the PyPDFLoader or similar document loaders to extract the content, which is then stored as a list of text documents for embedding and further processing.
Data Preparation Workflow
- Collect Documents: Gather text documents (e.g., PDFs).
- Load Text: Extract content using PyPDFLoader.
- Chunk Text: Split documents into smaller chunks with RecursiveCharacterTextSplitter.
- Generate Embeddings: Convert chunks to vector embeddings using OpenAIEmbeddings.
- Store Embeddings: Save embeddings in FAISS for fast retrieval.
- Extract Concepts: Use spaCy and LLMs for named entity and concept extraction.
- Build Knowledge Graph: Link document chunks based on similarity and shared concepts.
- Prepare Data: Organize the data for efficient querying and analysis.
Code Explanation
Mounting Google Drive
This code mounts Google Drive to Colab, allowing access to files stored in Drive. The mounted directory is /content/drive, enabling seamless file handling.
from google.colab import drive
drive.mount('/content/drive')
Installing Necessary Libraries
The code installs Python packages, which are essential for various tasks. The installation process adds langchain-community along with langchain-openai packages for language model usage and rank_bm25 alongside pymupdf and pypdf for PDF processing and Pydantic implements data validation and faiss-cpu enables quick similarity searches on extensive datasets.
!pip install -U langchain-community
!pip install langchain-openai
!pip install rank_bm25
!pip install pymupdf
!pip install pypdf
!pip install pydantic
!pip install faiss-cpu
Changing Directory in Google Colab
The command %cd /content/drive/MyDrive/New 90 Projects/generative_ai_project/GraphRAG changes the working directory to the specified folder in Google Colab.
%cd /content/drive/MyDrive/New 90 Projects/generative_ai_project/GraphRAG: Graph-Enhanced Retrieval-Augmented Generation
Importing Libraries for the Project
The code imports fundamental libraries which are necessary for the project implementation. The program loads natural language processing libraries such as NLTK and spaCy alongside the machine learning tools sklearn and numpy and the graph processing capabilities of networkx. LangChain fuels the system through document loading and embedding operations and retrieval tasks and the application benefits from thread pooling features and environment variable management functionalities.
# Import necessary libraries
import os
import sys
import heapq
import numpy as np
import spacy
import nltk
import networkx as nx
import matplotlib.pyplot as plt
import matplotlib.patches as patches
from typing import List, Tuple, Dict
from dotenv import load_dotenv
from tqdm import tqdm
from concurrent.futures import ThreadPoolExecutor, as_completed
from pydantic import BaseModel, Field
from sklearn.metrics.pairwise import cosine_similarity
# LangChain imports
from langchain.document_loaders import PyPDFLoader
from langchain.embeddings.openai import OpenAIEmbeddings
from langchain.vectorstores import FAISS
from langchain.text_splitter import RecursiveCharacterTextSplitter
from langchain.prompts import PromptTemplate
from langchain.retrievers import ContextualCompressionRetriever
from langchain.retrievers.document_compressors import LLMChainExtractor
from langchain.callbacks import get_openai_callback
from langchain_openai import ChatOpenAI
# NLTK imports
from nltk.stem import WordNetLemmatizer
from nltk.tokenize import word_tokenize
nltk.download('punkt', quiet=True)
nltk.download('wordnet', quiet=True)
# spaCy setup
from spacy.cli import download
from spacy.lang.en import English
# Set OpenAI API key (Replace YOUR_ACTUAL_API_KEY with your key)
%env OPENAI_API_KEY="ADD YOUR API KEY"
os.environ["KMP_DUPLICATE_LIB_OK"]="TRUE"
DocumentProcessor Class
The DocumentProcessor class serves as an interface for processing documents with embedding generation and document chunk similarity analysis.
__init__: During initialization, DocumentProcessor receives RecursiveCharacterTextSplitter to divide documents into chunks while using an OpenAIEmbeddings instance to embed these chunks.
process_documents: A document list is input into this method, which splits the documents into chunks while generating vectorized FAISS storage from embedded sections. When processing begins, it returns the separated documents together with the generated vector store.
create_embeddings_batch: This method embeds multiple text items in separate batches through OpenAI's framework. The function generates arrays containing embeddings that correspond to the provided input texts.
compute_similarity_matrix: This function computes cosine similarity between embedding vectors of input texts before returning a similarity matrix that depicts text-to-text relationships.
# Define the DocumentProcessor class
class DocumentProcessor:
def __init__(self):
"""
Initializes the DocumentProcessor with a text splitter and OpenAI embeddings.
Attributes:
- text_splitter: An instance of RecursiveCharacterTextSplitter with specified chunk size and overlap.
- embeddings: An instance of OpenAIEmbeddings used for embedding documents.
"""
self.text_splitter = RecursiveCharacterTextSplitter(chunk_size=1000, chunk_overlap=200)
self.embeddings = OpenAIEmbeddings()
def process_documents(self, documents):
"""
Processes a list of documents by splitting them into smaller chunks and creating a vector store.
Args:
- documents (list of str): A list of documents to be processed.
Returns:
- tuple: A tuple containing:
- splits (list of str): The list of split document chunks.
- vector_store (FAISS): A FAISS vector store created from the split document chunks and their embeddings.
"""
splits = self.text_splitter.split_documents(documents)
vector_store = FAISS.from_documents(splits, self.embeddings)
return splits, vector_store
def create_embeddings_batch(self, texts, batch_size=32):
"""
Creates embeddings for a list of texts in batches.
Args:
- texts (list of str): A list of texts to be embedded.
- batch_size (int, optional): The number of texts to process in each batch. Default is 32\.
Returns:
- numpy.ndarray: An array of embeddings for the input texts.
"""
embeddings = []
for i in range(0, len(texts), batch_size):
batch = texts[i:i+batch_size]
batch_embeddings = self.embeddings.embed_documents(batch)
embeddings.extend(batch_embeddings)
return np.array(embeddings)
def compute_similarity_matrix(self, embeddings):
"""
Computes a cosine similarity matrix for a given set of embeddings.
Args:
- embeddings (numpy.ndarray): An array of embeddings.
Returns:
- numpy.ndarray: A cosine similarity matrix for the input embeddings.
"""
return cosine_similarity(embeddings)
KnowledgeGraph Class: Building and Analyzing a Knowledge Graph
The KnowledgeGraph class creates and manages a graph of concepts derived from document content. It adds nodes for document splits, computes embeddings and extracts concepts using both spaCy and a large language model (LLM). The class also establishes connections (edges) between nodes based on the similarity of embeddings and shared concepts, with edge weights determined by both factors. This class helps structure and analyze information from a knowledge base, enabling efficient querying and exploration.