Question Answer System Training With Distilbert Base Uncased
Question Answering system built on Pegasus+SQuAD for accurate responses. Optimized for high accuracy and user experience across applications
$15 USD
$5.00 USD
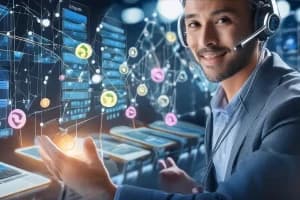
Project Outcomes
- Discover how to adjust and adapt pre-trained models like DistilBERT to question answering workloads.
- Get acquainted with one of the most used datasets in NLP: SQuAD (Stanford Question Answering Data set).
- Learn how to extract features from text data necessary for natural language processing models.
- Learn how to set up training loops with Hugging Face's Trainer API.
- Discover the essential skills for metrics such as accuracy and F1 score when testing a model.
- Share a deployed and trained model with the rest of the community through the Hugging Face Hub.
- Deal with big data using concepts of batch and pad.
- Learn how to use Google Colab's free GPU so that models train more quickly.
- Create a realistic artificial intelligence applied tool, something capable of answering questions based on a given context.
- The question-answering system built using DistilBERT can be deployed in customer service applications.
You might also like
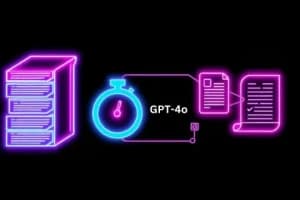
Optimizing Chunk Sizes for Efficient and Accurate Document Retrieval Using HyDE Evaluation
This project demonstrates the integration of generative AI techniques with efficient document retrieval by leveraging GPT-4 and vector indexing. It...
-with-dynamic-adjustment.webp&w=3840&q=75)
Corrective Retrieval-Augmented Generation (RAG) with Dynamic Adjustments
In the rapidly evolving field of artificial intelligence, the ability to retrieve accurate information and generate informed responses is paramount,...
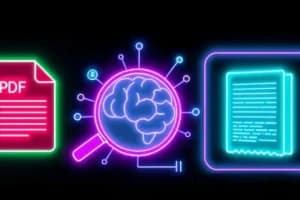
Enhancing Document Retrieval with Contextual Overlapping Windows
This project demonstrates a method to enhance document retrieval using contextually overlapping windows in a vector database. Adding surrounding context...
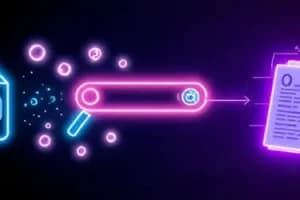
Document Augmentation through Question Generation for Enhanced Retrieval
This project focuses on document retrieval enhancement through text augmentation via question generation. The method aims to improve document search...