Credit Card Default Prediction Using Machine Learning Techniques
Preprocess data, engineer features, and apply models like Random Forest or XGBoost. Evaluate performance and use LIME/SHAP for interpretability.
$15 USD
$5.00 USD
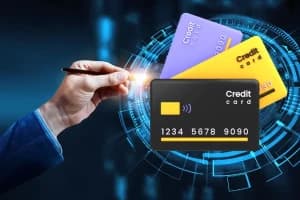
Project Outcomes
-
With the aid of SMOTE and downsampling, the model makes accurate predictions of customer defaults, which in turn assists financial institutions in risk reduction.
-
The prediction models can be explained through the use of SHAP and LIME techniques that ensure stakeholders understand how the model comes up with a decision.
-
The risk of default also helps businesses in estimating customer credit risk, which in turn informs their lending policies.
-
By managing retention risks and defaults, financial corporations can put in place measures for the customers at the risk of being high on the spot.
-
The model allows for loan approvals based on relevant data, which eliminates the need for manual assessments of borrowers and enhances efficiency.
-
Through such, financial service providers would focus on lending their resources to less risky clients avoiding high-risk clients.
-
If a business is able to correctly predict who will default on their debts, they can operate at a lower cost with regards to bad debt, chasing payment and writing off of loans.
-
The sermons based on predictions are more reliable, leading to business changes where they predict the customers are likely to produce positive results.
-
The model can be applied to a multitude of verticals in the finance sphere enabling banks, insurers, and lenders to predict defaults on scale.
-
Employing customer default predictive models helps banks in risk mitigation as it meets the demands of the ethical lending principle.