Context Enrichment Window Around Chunks Using LlamaIndex
Optimize document retrieval with AI using FAISS, OpenAI embeddings & context windows for smarter knowledge management & Q&A systems.
$20 USD
$10.00 USD
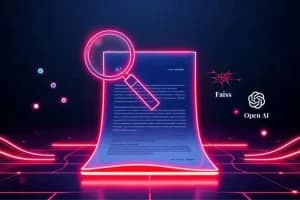
Project Outcomes
This project enhances AI-powered document retrieval by integrating LlamaIndex, FAISS, and OpenAI embeddings. It improves search accuracy by using sentence windows, ensuring contextually rich and relevant responses.
-
Enhances context-aware document retrieval by providing full context instead of isolated sentences.
-
Uses FAISS vector search for fast and scalable information retrieval.
-
Breaks down long PDFs into structured, searchable text chunks.
-
Allows customizable query processing for fine-tuned search results.
-
Improves AI-powered Q&A systems with more relevant, context-rich answers.
-
Demonstrates context-enriched search vs. standard retrieval for better accuracy.
-
Helps organizations manage internal documents and knowledge bases efficiently.
-
Optimizes data storage and retrieval using vector embeddings.
-
Enables quick document summarization for faster insights.
-
Can be integrated with LLMs like GPT-4o for AI-driven search applications.
You might also like
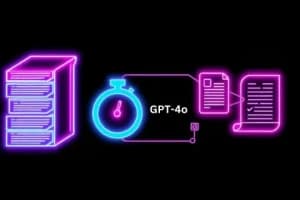
Optimizing Chunk Sizes for Efficient and Accurate Document Retrieval Using HyDE Evaluation
This project demonstrates the integration of generative AI techniques with efficient document retrieval by leveraging GPT-4 and vector indexing. It...
-with-dynamic-adjustment.webp&w=3840&q=75)
Corrective Retrieval-Augmented Generation (RAG) with Dynamic Adjustments
In the rapidly evolving field of artificial intelligence, the ability to retrieve accurate information and generate informed responses is paramount,...
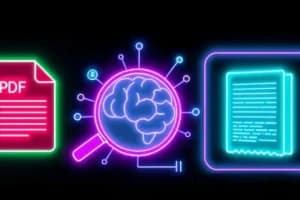
Enhancing Document Retrieval with Contextual Overlapping Windows
This project demonstrates a method to enhance document retrieval using contextually overlapping windows in a vector database. Adding surrounding context...
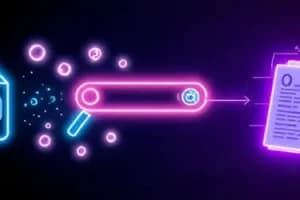
Document Augmentation through Question Generation for Enhanced Retrieval
This project focuses on document retrieval enhancement through text augmentation via question generation. The method aims to improve document search...