Blood Cell Classification Using Deep Learning
Our Blood Cell Classification project uses CNN, EfficientNetB4, and VGG16 models to correctly sort blood cell images. This reduces up and improves the accuracy of research, which helps doctors make better decisions.
Save $10
Limited Time Offer
$15 USD
$5.00 USD
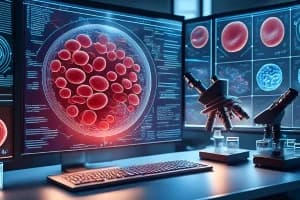
Project Outcomes
- The classification accuracy of EfficientNetB4 turned out to be the highest for automated blood cell analysis.
- As a result, correspondingly, the proposed model can help pathologists recognize cell types in little time and thus minimize their manual workloads.
- Blood cell differential helps in identifying diseases such as anemias, infections, and leukemia among other ailments.
- The automated system might also increase the diagnostic speed for use in high-throughput labs.
- When applied to the analysis of blood cells, the use of AI reduces the chances of human mistakes making the process more efficient.
- The feature of working with massive data is significant for large medical laboratories that analyze a few thousand samples daily.
- It shows how AI is used in doing everyday hematology work to ensure laboratories get efficient results out of blood samples.
- Some deep learning models can be used for diagnostics with no need for a specialist's contact; results can be delivered quickly to clinics, located in regions with limited access to specialists.
- A clinical project has demonstrated how the use of AI can enhance clinical processes to increase patient result delivery rate.
- This approach can therefore be easily extended to other related cell irregularities making it enormous in its diagnostic capability.
You might also like
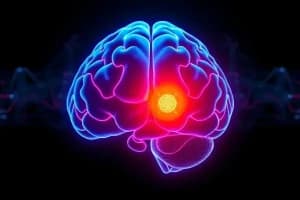
Image Segmentation using Mask R CNN with PyTorch
Mask-R-CNN is being employed to create a deep-learning model for detecting brain Tumors. The project's main focus is to automatically...
Deep Learning