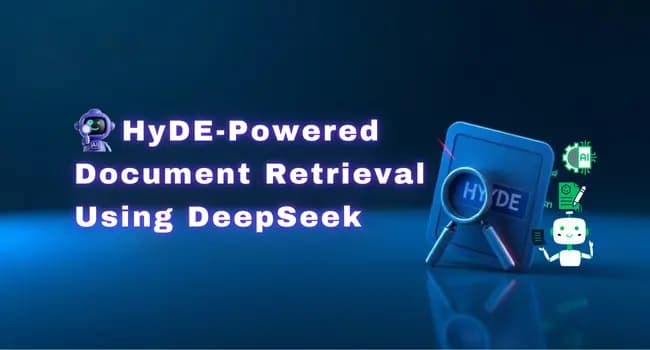
HyDE-Powered Document Retrieval Using DeepSeek
In this project, we're combining some exciting technologies such as FAISS, DeepSeek, LangChain and HuggingFace to develop an intelligent information retrieval system. The aim is to create a system that can efficiently load, process and store PDF documents, making it incredibly easy to search for and find relevant information. Whether you're posing a specific question or seeking context, the system will quickly generate responses and pull up the most pertinent documents.
Project Overview
Imagine having a bunch of PDF documents and then needing to pull out the exact answer for some specific inquiry. It is the LangChain system that loads and splits the documents and HuggingFace transforms the documents into embedded. Then comes DeepSeek, which creates a deep hypothetical answer to the question.
Once split and embedded, store the documents in FAISS, a quick vector store capable of efficiently searching for the most pertinent information. So, the answer to your query is generated by DeepSeek; along with that, important documents are also found with the use of FAISS. As a result, a smart and efficient system can be put in place for document analysis and query answering.
This system is all about finding accurate answers to a query by digging into the documents and clearing out all that mess of lines and pages written.
Prerequisites
- Python (Version 3.7 or higher)
- Google Colab (for easy access to GPU resources)
- Libraries:
- LangChain: For document processing and interaction with language models
- HuggingFace Transformers: For model handling and text embeddings
- FAISS: For efficient vector storage and similarity search
- PyMuPDF: For PDF loading and content extraction
- Sentence-Transformers: For text embedding generation
- Torch: For model inference and handling deep learning tasks
- Google Drive: To store and load PDF files
- Pre-trained Models (like DeepSeek or similar) for generating hypothetical answers and text generation
These tools and libraries will help you set up the system for loading documents, embedding them, generating answers and performing efficient document retrieval.
Approach
The approach of this project revolves around processing and embedding PDF documents into a FAISS vector store for fast and efficient similarity search. First, the PDF documents are loaded using LangChain's PyPDFLoader and split into smaller, manageable chunks using the RecursiveCharacterTextSplitter. These chunks are then embedded using HuggingFace's embeddings model, specifically designed to convert text into vector representations. Once embedded, the documents are stored in a FAISS vector store, enabling quick retrieval based on similarity to a given query. When a user submits a question, the system generates a hypothetical answer using the DeepSeek language model, which is then used to search for the most relevant documents in the FAISS vector store. The system returns both the generated hypothetical document and the retrieved documents, providing users with detailed, contextually relevant answers. This combination of deep learning models and efficient vector search techniques ensures a seamless, powerful solution for document analysis and query answering.
Workflow and Methodology
Workflow
- Step 1: Use LangChain's PyPDFLoader to load the PDF document.
- Step 2: Break the document into smaller sections with RecursiveCharacterTextSplitter to handle large texts more effectively.
- Step 3: Clean the text by removing unwanted characters, such as tabs, with a custom function.
- Step 4: Create embeddings for each text section using HuggingFace Embeddings.
- Step 5: Save the embeddings in a FAISS vector store for quick similarity searches.
- Step 6: For any given query, generate a hypothetical response using DeepSeek.
- Step 7: Conduct a similarity search in the FAISS vector store based on the generated response.
- Step 8: Retrieve the most relevant documents and present them alongside the hypothetical response.
Methodology
- Document Preprocessing: Utilize PyPDFLoader to extract text from PDF files and apply RecursiveCharacterTextSplitter to divide the text into smaller, meaningful segments.
- Text Embedding: Transform the document segments into vector embeddings using a pre-trained HuggingFace model that captures the semantic meaning of the text.
- Vector Store: Save the embeddings in a FAISS vector store, enabling efficient retrieval of similar documents based on query relevance.
- Query Answering: When a user submits a query, employ DeepSeek (or another suitable LLM) to create a hypothetical answer, which is then used to find the most relevant documents in the vector store.
- Similarity Search: Leverage FAISS to conduct a similarity search and obtain the top-k most relevant documents that correspond to the hypothetical answer or query.
- Result Presentation: Present the generated hypothetical answer alongside the retrieved documents for comprehensive context.
Data Collection and Preparation
Data Collection
Gather all PDF files that contain the relevant content for processing. Store them in an accessible location, such as Google Drive, for easy access to the code.
Data Preparation Workflow
- Use LangChain's PyPDFLoader to extract raw text from PDFs.
- Split the text into smaller chunks using RecursiveCharacterTextSplitter (e.g., 1000 characters).
- Clean the text by removing unwanted characters (e.g., tabs).
- Use HuggingFace embeddings (e.g., all-MiniLM-L6-v2) to convert text into vector representations.
- Store the embeddings in a FAISS vector store for fast search.
- The system is ready to return relevant documents based on user queries using FAISS.
Code Explanation
STEP 1:
Installation of Required Libraries
This code installs essential libraries for natural language processing and document processing. It includes LangChain for managing language models, sentence-transformers for embeddings, PyMuPDF for working with PDFs and FAISS for similarity search. Additionally, OpenAI and Cohere APIs are installed for language model integration.
!pip install langchain langchain-community langchain-openai langchain-cohere sentence-transformers faiss-cpu PyMuPDF rank-bm25 openai transformers torch accelerate
!pip install pypdf
Importing Necessary Libraries
This code imports libraries for document processing, text splitting and embeddings. It uses PyPDFLoader from LangChain to load PDF files, RecursiveCharacterTextSplitter to split text and HuggingFaceEmbeddings for embeddings. Additionally, it imports FAISS for vector storage, Hugging Face's AutoModelForCausalLM for language models and pipeline for task-specific pipelines. Torch is used for model handling.
import os
import textwrap
import numpy as np
from typing import List
from langchain.document_loaders import PyPDFLoader
from langchain.text_splitter import RecursiveCharacterTextSplitter
from langchain.embeddings import HuggingFaceEmbeddings # Using Hugging Face instead of OpenAI
from langchain.vectorstores import FAISS
from transformers import AutoModelForCausalLM, AutoTokenizer, pipeline
import torch
STEP 2:
Mounting Google Drive
This code mounts Google Drive to the Colab environment, allowing access to files stored on the drive. The drive is mounted at /content/drive, enabling file interactions within the Colab notebook.
from google.colab import drive
drive.mount('/content/drive')
Setting the PDF File Path
This code assigns the path of the PDF file (tesla.pdf) stored on Google Drive to the pdf_path variable. The path can be updated if a different file is needed.
pdf_path = "/content/drive/MyDrive/tesla.pdf" # Change this if needed
Loading the Pre-trained Model
This code sets the model name and loads the tokenizer and model for inference. It uses the AutoTokenizer and AutoModelForCausalLM from Hugging Face, loading the model DeepSeek-R1-Distill-Qwen-1.5B. It creates a text generation pipeline using the loaded model, optimized for inference with torch.float16.
MODEL_NAME = "deepseek-ai/DeepSeek-R1-Distill-Qwen-1.5B" # Change to DeepSeek if needed
# Load tokenizer & model
tokenizer = AutoTokenizer.from_pretrained(MODEL_NAME)
model = AutoModelForCausalLM.from_pretrained(
MODEL_NAME, device_map="auto", torch_dtype=torch.float16
)
# Create inference pipeline
llm_pipeline = pipeline("text-generation", model=model, tokenizer=tokenizer)
STEP 3:
Replacing Tabs with Spaces in Documents
This function takes a list of documents and replaces all tab characters (\t) with spaces in the content of each document. It iterates through the documents, modifies their page_content and returns the updated list.