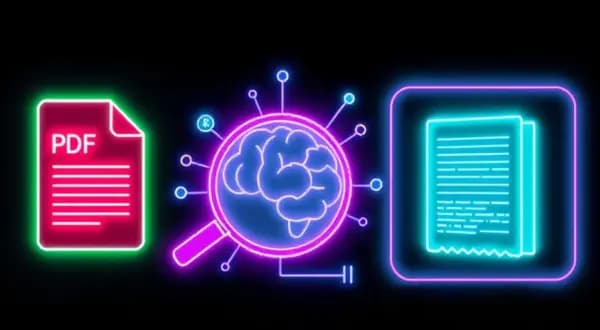
Enhancing Document Retrieval with Contextual Overlapping Windows
This project demonstrates a method to enhance document retrieval using contextually overlapping windows in a vector database. Adding surrounding context to retrieved text chunks improves the coherence and completeness of the information. The approach uses PDF processing, text chunking, and FAISS with OpenAI embeddings to create a vector store. A custom retrieval function fetches relevant chunks with added context, offering a better alternative to traditional vector search methods that often return isolated, context-lacking information.
Project Overview
This project focuses on enhancing document retrieval by incorporating contextually overlapping windows in a vector database. Traditional vector search methods often return isolated chunks of text that may lack sufficient context, making it harder to understand the information. This technique addresses this issue by adding surrounding context to the retrieved chunks, improving the coherence and completeness of the results.
The project involves PDF processing, which divides documents into manageable text chunks. These chunks are stored in a vector store using FAISS and OpenAI embeddings to facilitate fast retrieval. A custom retrieval function is then used to fetch relevant chunks and their surrounding context. The effectiveness of this approach is compared with standard retrieval methods, offering a more comprehensive and accurate search experience.
Prerequisite
- Familiarity with Python
- Knowledge of text chunking and contextual information retrieval
- Experience with Colab Notebooks for project development
- Basic understanding of document retrieval and vector databases,
- Libraries: Python, FAISS (for vector search and indexing), OpenAI embeddings (for text embeddings), NumPy, Pandas, PyPDF2, and LangChain.
- Basic knowledge of embedding generation and usage with FAISS.
Approach
The approach involves improving document retrieval by incorporating contextually overlapping windows. First, documents are processed using PDF extraction techniques like PyPDF2 to break them down into manageable text chunks. These chunks are then stored in a vector database using FAISS for efficient search and retrieval. To enhance the search results, OpenAI embeddings are used to generate vector representations of the text chunks, ensuring semantic accuracy. When a query is made, a custom retrieval function fetches the relevant text along with its surrounding context, creating a more comprehensive and coherent response. This method is compared against traditional retrieval techniques, highlighting improvements in context and results in completeness.
Workflow and Methodology
Workflow
- Extract text from PDFs using PDF processing libraries (e.g., PyPDF2)
- Divide the extracted text into smaller chunks for easier processing.
- Generate embeddings for each text chunk using OpenAI embeddings.
- Store the embeddings in a FAISS vector database for efficient searching.
- Create a custom retrieval function that retrieves text chunks along with their surrounding context.
- Compare results from standard retrieval and contextual retrieval to evaluate improvements in coherence and completeness.
Methodology
- Text Processing: Use PDF extraction to parse documents and convert them into text chunks.
- Embedding Generation: Apply OpenAI embeddings to generate vector representations of the text chunks.
- Vector Search: Store these embeddings in a FAISS database for fast retrieval based on similarity.
- Contextual Retrieval: Implement a custom retrieval function that fetches relevant chunks and includes their surrounding context to provide more complete answers.
- Evaluation: Compare the new method with traditional retrieval to assess contextual understanding and search accuracy improvements.
Data Collection and Preparation
Data Collection
The data used in this project consists of PDF documents called Climate_Change.pdf, which are stored in a specific directory. The PDF files contain textual information that is extracted and processed. The extraction process involves using libraries like PyPDF2 to pull out the text content from these documents.
Data Preparation Workflow:
- Extract text from PDFs using PyPDF2 or pdfplumber.
- Clean the text by removing unnecessary characters and formatting.
- Split the text into smaller chunks.
- Generate embeddings for each chunk using OpenAI embeddings.
- Store the embeddings in a FAISS vector database.
- Group chunks with surrounding context for more coherent retrieval.
- Validate the data for accuracy and correct embedding storage.
Code Explanation
Mounting Google Drive
This code mounts Google Drive to Colab, allowing access to files stored in Drive. The mounted directory is /content/drive, enabling seamless file handling.
from google.colab import drive
drive.mount('/content/drive')
Installing Necessary Packages
These commands install several useful Python packages: LangChain-OpenAI connects your code to OpenAI's language models and LangChain-community adds extra community tools, sentence_transformers converts text into numerical vectors for analysis, DuckDuckGo lets you search the internet using DuckDuckGo, PyPDF2 helps you work with PDF files, tiktoken tokenizes text for processing, and faiss-cpu enables fast similarity searches in large datasets.
!pip install langchain-openai
!pip install langchain-community
!pip install sentence_transformers
!pip install -U duckduckgo-search
!pip install PyPDF2
!pip install tiktoken
!pip install faiss-cpu
Library Import and Key Setup
This code imports necessary libraries for handling PDFs, JSON data, system paths, and interfacing with OpenAI and other tools; it then retrieves an API key from either Colab or the system environment and sets it, appending a parent directory to the system path and confirming successful setup with a print message.
import os
import sys
import json
import PyPDF2
from typing import List, Tuple
from langchain.schema import Document
from langchain.vectorstores import FAISS
from langchain.prompts import PromptTemplate
from langchain_openai import ChatOpenAI
from langchain_core.pydantic_v1 import BaseModel, Field
from langchain.tools import DuckDuckGoSearchResults
from langchain.embeddings import OpenAIEmbeddings
import warnings
warnings.filterwarnings("ignore")
try:
from google.colab import userdata
api_key = userdata.get("OPENAI_API_KEY")
except ImportError:
api_key = None # Not running in Colab
if not api_key:
api_key = os.getenv("OPENAI_API_KEY")
if api_key:
os.environ["OPENAI_API_KEY"] = 'ADD YOUR OPENAI_API_KEY'
else:
raise ValueError(" OpenAI API Key is missing\! Add it to Colab Secrets or .env file.")
sys.path.append(os.path.abspath(os.path.join(os.getcwd(), '..')))
print("OPENAI_API_KEY setup completed successfully!")
File Path Setup
This code sets the variable path to the specific location of the "Climate_Change.pdf" file in Google Drive, indicating where the file is stored for later access and processing in the project.
path = "/content/drive/MyDrive/Context Enrichment Window Around Chunks Using LlamaInde/Climate_Change.pdf"
Read PDF Function
This function opens a PDF file in binary mode, reads text from each page using PyPDF2, concatenates all the extracted text into one single string, and returns that string for further use.
def read_pdf_to_string(pdf_path):
"""Reads a PDF file and returns its content as a string.
Args:
pdf_path (str): The path to the PDF file.
Returns:
str: The content of the PDF file as a string.
"""
with open(pdf_path, 'rb') as pdf_file:
pdf_reader = PyPDF2.PdfReader(pdf_file)
num_pages = len(pdf_reader.pages)
text = ""
for page_num in range(num_pages):
page = pdf_reader.pages[page_num]
text += page.extract_text()
return text
PDF Content Extraction
This code calls the read_pdf_to_string function using the specified file path and stores the extracted text from the PDF into the variable content, making the PDF’s text available for further processing.